Artificial intelligence (AI) is the talk of the town these days, and it plays a significant role in every industry. This technology, however pervasive is still in its infancy and it’s impact is still indeterminant. Calvin D. Lawrence, Distinguished Engineer at IBM is concerned about ethical issues of AI in the healthcare sector when proper guardrails and governance techniques have not been considered.
Ethical AI Considerations
Social determinants like race, age, gender, income, zip code, and education have historically been used to determine healthcare outcomes. These social determinants often make their way into decision points used by AI algorithms. Despite race being a socio-political categorization system without a biological and scientific basis, race has historically. It continues to play a role in medical teaching and clinical decision-making within health care. Race permeates clinical decision-making and treatment in multiple ways, including:
- Through providers’ attitudes and implicit biases.
- Disease stereotyping and clinical terminology.
- Clinical algorithms, tools, and treatment guidelines.
Current engineering practices, such as restricting specific fields such as race or gender from the data and limiting the issues to engineering and technical matters, won’t solve the problem alone. If the world looks a certain way, that will be reflected in the data, either directly or through proxies, and thus in the decisions produced from the data.
Calvin Lawrence, an expert, addresses such topics as AI bias and how race based medicine continue to enable stereotypical-driven medical and healthcare outcomes.
Using Guardrails to Prevent Unintended Outcomes
It is an undeniable fact that bias in the AI always have repercussions for the patient and data provider. The irony is that the company seldom suffers from bias at the same level as those who are unfairly targeted. Using guardrails to prevent undesired results must be the order of the day, as it prevents data inaccuracies and privacy breaches.
Why Should Corporations Care
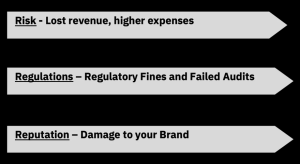
Why People of Color Care
Patients may not receive the care they need if healthcare decision points offered by algorithms are biased. When there are actual underlying health issues that need to be treated, doctors may automatically assign symptoms to a problem with weight, race, or gender. When thinking about healthcare bias, many individuals of color should be concerned about the following three examples:
-
- People of Color often get inferior healthcare services – Bias-driven discriminatory practices and policies not only negatively affect our care and our access to proper care but also limit the diversity of the healthcare workforce, often leading to inequitable distribution of research funding and can hinder career advancement. That means we are less likely to see doctors and clinical staff that looks like us.
- People of Color are often misdiagnosed – Studies show that some clinical algorithms used by hospitals to decide which patients need care can be racially bias.
- People of Color are not properly represented in clinical trials – For minority populations, overcoming historical stigma is a big concern and is probably one of the main causes of the low participation rates. The clinical studies serve as reminders of how ethics, race, and medicine frequently interact negatively and foster mistrust in the Black community. For many healthcare algorithms, clinical trial data is a common source of information.
Best Practices for Eliminating Bias in Healthcare
Using Guardrails to Prevent Unintended Outcomes against People of Color
It is an undeniable fact that bias in the AI always have repercussions for the patient and data provider. The irony is that the company seldom suffers from bias, which is why it must be held accountable for the bias and its resulting impact on the patient. Using guardrails to prevent undesired results must be the order of the day, as it prevents data inaccuracies and privacy breaches. Some guardrails to consider are:
- Diversity on the data science team – Ensuring that your team as well as training data properly represents all aspects of your intented outcome.
- Policy and Legislation – The advocation for and the adherence to corporate, local, state and federal legislation that ensures bias-free AI solutions
- Access the Impact prior to deployment – Assessing the impact of what could go wrong when the model fails prior to deployment
- Model Explainability – How did AI arrive at its outcome? How and When would the outcome be different
- Certification & Conformity – Ensuring that a given system is legally compliant, ethically sound, and technically robust
Final Word
Artificial Intelligence is taking the world by storm. From the most basic daily operations to complex technological industries, you are bound to witness astonishing technological innovations regularly taking place. Automation and machine learning are evolving with the help of Big Data to counter processes across industries that help reduce the wastage of resources, optimize and automate processes, simplifying the lives of business owners and their consumers.
About Calvin Lawrence
Calvin Lawrence is a Distinguished Engineer at IBM specializing in AI and ensuring its responsible usage. He is also passionate about the fair and ethical use of technology, and his recent book Hidden in White Sight: How AI Empowers and Deepens Systemic Racism has captured the hearts and minds of AI and socially conscious enthusiasts across the globe. PREORDER YOUR COPY TODAY